Merchants face special challenges when operating large affiliate marketing programs: rogue affiliates can claim to refer users who would have purchased from those merchants anyway. In particular, rogue “cookie-stuffer” affiliates deposit cookies invisibly and unrequested — knowing that a portion of users will make purchases from large merchants in the subsequent days and weeks. This tactic is particularly effective in defrauding large merchants: the more popular a merchant becomes, the more users will happen to buy from that merchant within a given referral period.
To cookie-stuff at scale, an attacker needs a reliable and significant source of user traffic. In February we showed a rogue affiliate hacking forum sites to drop cookies when users merely browse forums. But that’s just one of many strategies. I previously found various cookie-stuffing on sites hoping to receive search traffic. In a 2009 complaint, eBay alleges that rogue affiliates used a banner ad network to deposit eBay affiliate cookies when users merely browsed web pages showing certain banner ads. See also my 2008 report of an affiliate using Yahoo’s Right Media ad network to deposit multiple affiliate cookies invisibly — defrauding security vendors McAfee and Symantec.
As the eBay litigation indicates, display advertising networks can be a mechanism for cookie-stuffing. Of course diligent ad networks inspect ads and refuse cookie-stuffers (among other forms of malvertising). So we were particularly surprised to see Google AdSense running ads that cookie-stuff Amazon.
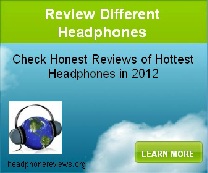
This innocuous-looking banner ad sets Amazon Associates cookies invisibly.
The Imgwithsmiles attack
We have uncovered scores of web sites running the banner ad shown at right. On 40 sites, on various days from February 6 to May 2, our crawlers found this banner ad dropping Amazon Associates affiliate cookies automatically and invisibly. All 40 sites include display advertising from Google AdSense. Google returns a Flash ad from Imgwithsmiles. To an ordinary user, the ad looks completely innocuous — the unremarkable “review different headphones” image shown at right. However, the ad actually creates an invisible IMG (image) tag loading an Amazon Associates link and setting cookies accordingly. Here’s how:
First, the ad’s Flash code creates an invisible IMG tag (10×10 pixels) (yellow highlighting below) loading the URL http://imgwithsmiles.com/img/f/e.jpg (green).
function Stuff() {
if (z < links.length) {
txt.htmltext = links[z];
z++;
return(undefined);
}
clearinterval(timer);
}
links = new array();
links[0] = "<img src="http://imgwithsmiles.com/img/f/e.jpg" width="10" height="10"/>";z = 0;timer = setinterval(Stuff, 2000);
While /img/f/e.jpg features a .jpg extension consistent with a genuine image file, it is actually a redirect to an Amazon Associates link. See the three redirects preserved below (blue), including a tricky HTTPS redirect (orange) that would block many detection systems. Nonetheless, traffic ultimately ends up at Amazon with an Associates tag (red) specifying that affiliate charslibr-20 is to be paid for these referrals.
GET /img/f/e.jpg HTTP/1.0
Accept: */*
Accept-Language: en-US
Referer: http://pagead2.googlesyndication.com/pagead/imgad?id=CICAgICQvuXgahDQAhiYAjII3bQHU19r_Isx-flash-version: 10,3,183,7User-Agent: Mozilla/4.0 (compatible; MSIE 8.0; ...)Host: imgwithsmiles.comConnection: Keep-AliveHTTP/1.1 302 Moved TemporarilyDate: Wed, 02 May 2012 19:56:59 GMTServer: Apache/2.2.21 (Unix) mod_ssl/2.2.21 OpenSSL/0.9.8e-fips-rhel5 mod_bwlimited/1.4
X-Powered-By: PHP/5.2.17
Expires: Thu, 19 Nov 1981 08:52:00 GMT
Cache-Control: no-store, no-cache, must-revalidate, post-check=0, pre-check=0
Pragma: no-cache
Set-Cookie: PHPSESSID=174272468a212dd0862eabf8d956e4e0; path=/
Location: https://imgwithsmiles.com/img/kick/f/e.jpg
Content-Length: 0
Keep-Alive: timeout=5, max=100
Connection: Keep-Alive
Content-Type: text/html-
HTTPS redirect decoded via separate manual request
GET /img/kick/f/e.jpg HTTP/1.1
Accept: text/html, application/xhtml+xml, */*
Accept-Language: en-US
User-Agent: ...
Accept-Encoding: gzip, deflate
Host: imgwithsmiles.com
Connection: Keep-AliveHTTP/1.1 302 Moved Temporarily
Date: ...
Server: Apache/2.2.21 (Unix) mod_ssl/2.2.21 OpenSSL/0.9.8e-fips-rhel5 mod_bwlimited/1.4
X-Powered-By: PHP/5.2.17
Location: http://imgwithsmiles.com/img/t/f/e.jpg
Content-Length: 0
Connection: close
Content-Type: text/html-GET /img/t/f/e.jpg HTTP/1.0
Accept: */*
Accept-Language: en-US
x-flash-version: 10,3,183,7
User-Agent: Mozilla/4.0 (compatible; ...)
Connection: Keep-Alive
Host: imgwithsmiles.com
Cookie: PHPSESSID=174272468a212dd0862eabf8d956e4e0HTTP/1.1 302 Moved TemporarilyDate: Wed, 02 May 2012 19:56:59 GMT
Server: Apache/2.2.21 (Unix) mod_ssl/2.2.21 OpenSSL/0.9.8e-fips-rhel5 mod_bwlimited/1.4
X-Powered-By: PHP/5.2.17
Expires: Thu, 19 Nov 1981 08:52:00 GMT
Cache-Control: no-store, no-cache, must-revalidate, post-check=0, pre-check=0
Pragma: no-cache
Location: http://www.amazon.com/gp/product/B002L3RREQ?ie=UTF8&tag=charslibr-20
Content-Length: 0
Keep-Alive: timeout=5, max=99
Connection: Keep-Alive
Content-Type: text/html
If a user happens to make a purchase from Amazon within the subsequent 24 hours, Amazon will pay a commission to this affiliate — even though the affiliate did nothing at all to cause or encourage the user to make that purchase.
Does Amazon know?
The available information does not reveal whether or not Amazon knew about this affiliate’s practices. Nor can we easily determine whether, as of the May 2, 2012 observations presented above, this affiliate was still in good standing and receiving payment for the traffic it sent to Amazon.
On one hand, Amazon is diligent and technically sophisticated. Because Amazon runs one of the web’s largest affiliate programs, Amazon is necessarily familiar with affiliate fraud. And Amazon has ample incentive to catch affiliate fraud: Every dollar paid to fraudulent affiliates is money completely wasted, coming straight from the bottom line.
On the other hand, we have observed this same affiliate cheating Amazon for three months nonstop. All told, we’ve seen this affiliate rotating through 49 different Associates IDs. If Amazon had caught the affiliate, we would have expected the affiliate to shift away from any disabled affiliate accounts, most likely by shifting traffic to new accounts. Of the 28 Associates IDs we observed during February 2012, we still saw 6 in use during May 2012 (month-to-date) — suggesting that while Amazon may be catching some of the affiliate’s traffic, Amazon probably is not catching it all.
A further indication of the affiliate’s earnings comes from the affiliate’s willingness to incur out-of-pocket costs to buy media (AdSense placements from Google) with which to deliver Amazon cookies. As best we can tell, Amazon is the affiliate’s sole source of revenue. Meanwhile, the affiliate must pay Google for the display ad inventory the affiliate receives. These direct incremental costs give the affiliate a clear incentive to cease operation if it concludes that payment from Amazon will not be forthcoming. From the affiliate’s ongoing actions we can infer that the affiliate finds this scheme profitable — that its earnings to date have exceeded its expenses to date.
How profitable is this affiliate’s attack? Conservatively, suppose 40% of users are Amazon shoppers and make an average of four purchases from Amazon per year. Then 0.4*4/365=0.44% of users are likely to make purchases from Amazon in any given 24-hour period. Suppose the affiliate buys 1,000,000 CPM impressions from Google. Then the affiliate will enjoy commission on 0.44%*1,000,000=4,384 purchases. At an average purchase size of $30 and a 6.5% commission, this would be $8,547 of revenue per million cookie-stuffing incidents. How much would the affiliate have to pay Google for 1,000,000 CPM impressions? We’ve seen this affiliate on a variety of sites, but largely sites in moderate to low-priced verticals. At $2 CPM, the affiliate’s costs would be $2,000 — meaning the affiliate would still be slightly profitable even if Amazon caught 3/4 of its affiliate IDs before the first payment!
We alerted our contact at Amazon Associates to our observations. We will update this post with any information Amazon provides.